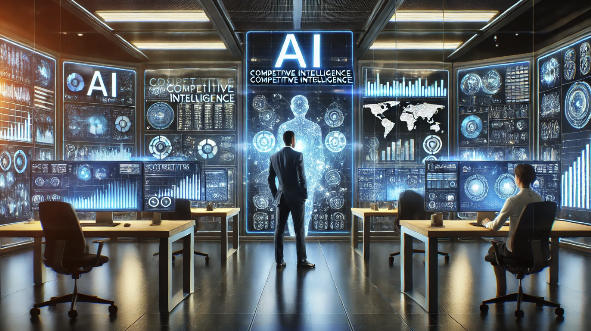
On November 14th, I had the opportunity to attend a day of conferences focused on information and artificial intelligence, organized by Archimag. During the event, I was invited to speak and share my perspective as an information professional on Artificial Intelligence, its contribution to the field of competitive intelligence, and its potential limitations.
Although artificial intelligence has been present for quite some time in commercial offerings and software functionalities related to information professions (such as documentation, competitive intelligence, knowledge management, and countless others), its value, profitability, and performance have often been questionable and highly variable depending on its application.
The advent of OpenAI and the emergence of numerous LLMs (Large Language Models) have accelerated innovation in artificial intelligence, particularly in generative aspects. Beyond the impressive capabilities of these new tools, I aimed to highlight the key functionalities that enable these tools to address the specific challenges faced by competitive intelligence professionals.
Artificial Intelligence and Productivity gains
International Competitive Intelligence
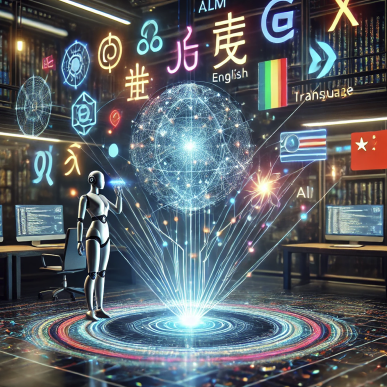
Many intelligence professionals work with international sources, including some in languages they may have little or no proficiency in. By integrating translation functions, AI tools complement the existing array of translation tools such as Google Translate, DeepL, and Systran (which, incidentally, is now under the umbrella of ChapsVision, which has also acquired Qwam and Bertin IT, including the AMI solution, a well-known provider among intelligence professionals).
However, at this stage, the quality improvement in translation brought by LLMs is not particularly striking. Given the relative infancy of LLMs and their ability to self-improve, we can reasonably expect quality improvements over time.
It should be noted that both traditional machine translation tools (Google Translate, DeepL, Systran) and LLMs offer API access. This allows their integration without requiring direct interaction with their interfaces, enabling seamless incorporation into user environments—for example, as implemented by Cikisi in a way that is completely transparent to intelligence professionals.
The advantage of integrating an LLM-based AI versus older translation tools lies in the following points:
- If the translation quality of AI is currently more or less equivalent to that of existing tools, significant progress can be expected in the coming years, given the rapid pace of improvement in LLMs over just a few months, particularly in their broader generative functions.
- Integrating AI into a process, or even directly into a competitive intelligence software, allows reliance on a single technology that can also offer additional functionalities such as document summarization, analytical support, or exploration of document corpora. This makes the cost of integration easier to amortize more quickly. Moreover, depending on the business models of LLMs, economies of scale can be achieved by using them for a higher number of queries.
The main drawback I see is the same as with automatic translation tools in general. While non-human translation provides a sufficient initial level of quality to determine whether a piece of information is interesting or not, it can sometimes be challenging to grasp nuances or avoid misunderstandings when sentence structures are somewhat ambiguous.
Finally, LLMs and their capabilities are also tied to the datasets and sources used to train them, both before their launch and throughout their lifecycle. LLM-based AIs often rely heavily on English-language content, leading to a cultural bias in their understanding of content and their generative capabilities.
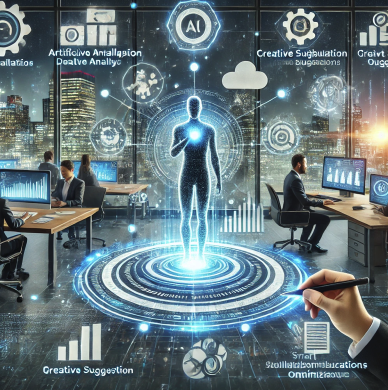
Information Synthesis
“Regarding first-level information synthesis in exploratory phases, LLMs have so far had no equivalent and provide a significant level of quality and considerable time savings in certain configurations.
As with all software tools (and indeed all forms of intelligence, human or artificial), the principle of ‘trash in, trash out’ applies. If the AI is fed with heterogeneous document corpora of varying quality, potentially including false information, spam, or advertisements, one should not expect a high-quality synthesis. However, for an intelligence professional using generative tools based on LLMs with pre-cleaned and carefully selected document corpora, the AI can save valuable time by producing a high-quality synthesis.
Through successive queries and the ability of AIs to engage in dialogue with the intelligence professional, it becomes possible to refine the synthesis, target specific areas, filter content, and explore certain aspects more deeply through a series of prompts—all within a short amount of time.”
Using LLMs will prove particularly relevant for topics where the intelligence professional has only a superficial understanding, allowing them to quickly outline the key aspects and get to the core of the subject.
For a more experienced competitice intelligence professional on a given topic, the productivity gains will be most notable during the writing phases. This gain will be even greater because, in many cases, the professional will not need to verify the information underlying the synthesis, as they are already well-versed in the subject and capable of identifying and avoiding potential misinterpretations by the AI.
However, a major risk associated with AI in document synthesis is that the synthesized content often strays significantly from the original content, making verification of the synthesis a time-consuming process.
Additionally, AI suffers from the same cognitive biases linked to conditioning. The more an AI is exposed to a particular piece of information, the more, like the human brain, it will naturally tend to view it as significant and assign it undue importance in the synthesis. This can lead to overvaluing spam or advertorial content that saturates media, underscoring the importance of applying AI to well-curated corpora.
Another problematic issue is that relying consistently on AI for creating syntheses may cause intelligence professionals to gradually lose touch with the original content. This could lead to a decline in their sectoral knowledge, leaving them dependent on AI-generated pre-digested content and, eventually, unable to assess its credibility or quality.
Information analysis functions
One of the major strengths of AI in analysis is its ability to ingest and process a very large number of documents.
An analytical bias often arises in the early stages of analysis, particularly during the creation of the corpus that will serve as the basis for the analysis. In this specific case, instead of introducing a bias, AI can help mitigate the inherent bias caused by the selection process performed by the intelligence professional or analyst, who, due to limited assimilation capacity, may be compelled to reduce the volume of documents used for the analysis.
In this scenario, and as mentioned earlier, AI may have a tendency to prioritize strong and recurring signals within the corpus. However, leveraging conversational techniques with the AI should enable the elimination of dominant signals and allow for the generation of sub-analyses that highlight emerging signals or weak signals.”
AI and copyright
Content that is sufficiently rich and detailed for intelligence professionals and their users while remaining compliant with copyright laws.
The days when librarians or even intelligence professionals manually wrote summaries of articles are long gone. They no longer have the time or budgets to allocate to fully rewriting shared content, which used to help strike that delicate balance between providing valuable information and ensuring compliance.
The costs associated with redistributing full-text articles to compensate authors are still based on business models that have seen little evolution in recent years, unlike the music industry, which has transitioned to an unlimited subscription model widely adopted by musicians.
AI offers real solutions to these challenges by providing functionalities such as:
- Translation
- Rewriting, synthesis, and abstracts that can replace summaries
- Creation of visuals
These synthesis tools are currently being integrated or have already been integrated into various competitive intelligence software solutions, such as Curebot, Cikisi, and Sindup.
However, I emphasize the importance of exercising caution with this use case.
Indeed, several publications highlight the tendency of LLMs to sometimes reproduce significant portions of original articles verbatim, thereby exposing intelligence professionals to copyright infringements, often without their awareness.
Developers of LLMs have recently been focusing on training their models to better respect copyright laws, especially as legal actions by rights holders or their representatives are becoming increasingly frequent.
AI integratyion within Competitive Intelligence software
As mentioned, AI can bring real efficiency to the daily tasks of an intelligence professional. However, one essential prerequisite is that it must be fully integrated into the competitive intelligence workflow.
The success of historical intelligence platforms such as Digimind, KB Crawl, AMI, or Sindup lies in their ability to integrate the various phases of the information cycle (collection, processing, dissemination, and sometimes analysis, although this latter phase is mostly conducted outside these platforms) within a single interface and tool.
AI becomes truly valuable when it is integrated ergonomically, even transparently, into these tools to maximize efficiency gains.
The challenge, however, is that some of these intelligence technologies are somewhat outdated, and their various versions and updates have not necessarily facilitated the integration of third-party technologies or the use of APIs.
Moreover, when intelligence platform providers integrate features based on LLMs, they often limit the choice of models. As a result, users and clients of an intelligence platform cannot select between models such as OpenAI, Copilot, or others.
The integration of AI technologies into competitive and strategic intelligence platforms also raises the question of confidentiality. Using an API means that data will be sent to a third-party solution outside the intelligence platform, processed by the AI, and returned as the expected result—be it a translation, summary, or analysis.
As a result, intelligence software providers primarily choose to rely on open-source AIs such as BERT or Llama, for example.
IA et Business Model
AI will deliver its full value when it is fully integrated into tools and processes. This requires software development costs, human time, training expenses, and a certain inertia—like with any new tool—before reaching peak efficiency.
This represents a significant investment for both companies and software providers.
However, with only a few years of existence (or at least market deployment), the business model of these providers remains unclear.
For now, each LLM is striving to carve out a share of the market using diverse strategies: some, like OpenAI with its GPT-4 or GPT-4.1, are playing the omniscience card by attempting to offer services related to image generation, text generation, and information retrieval. Others are specializing to quickly demonstrate their effectiveness in specific market segments by focusing their AI training and enabling rapid knowledge acquisition in a limited spectrum, such as Yseop in the pharmaceutical sector.
But what about tomorrow ?
In a world where sustainability and ecology are becoming increasingly important, what can we expect in the future regarding regulatory constraints and their impact on the cost of these technologies?
Moreover, once the market has consolidated, how can we not foresee a significant price increase, especially once companies become dependent on these technologies after investing substantial amounts in their integration?
Unfortunately, the brief history of digital technology has shown us that the two most viable models so far have been:
- The shift to a paid model to cover fixed and variable costs, which is FAR from being the case today for OpenAI, for instance. OpenAI is projecting a net loss of $5 billion by the end of this year, clearly indicating that the current pricing does not reflect the true cost of the service.
- The adoption of an advertising model, which seems unlikely, at least for professional use cases.
The increase in energy costs, which are heavily consumed by these technologies, combined with increasingly stringent environmental constraints, the current underpricing of subscriptions, and the massive investments required for ongoing innovation, all point to a substantial rise in subscription fees (already +10% for ChatGPT in 2024).
Finally, unlike most software, where once the costs of development, maintenance, and updates are absorbed, the marginal cost per user remains limited, the cost structure for AI solutions is very different. Each prompt consumes significant energy, resulting in high variable costs that are proportional to usage. This creates a dangerous equation: the more users are trained, the more efficient the solution becomes, the more a user maximizes their subscription, generating high variable costs that limit the margins on each subscription.
This explains why some companies are turning to open-source AI solutions, which may be less performant than proprietary LLM market leaders but offer a way to mitigate the risk of financial overreach.
AI and authors
I will conclude by addressing two additional points.
First, the issue of intellectual property. Until now, AI models have largely been trained using online content that is publicly accessible. While these models do not redistribute this content in its original form, they exploit original content without compensating the authors, to generate their own content and derive profit from it.
It is therefore understandable that authors are beginning to push back against these new technologies, which they see as parasitic—profiting from their work to generate revenue without ensuring fair compensation for those who made these AIs what they are.
For example, The Times has filed lawsuits against OpenAI and Microsoft AI, and author societies have criticized LLM developers for failing to respect copyright laws.
Lawsuits are multiplying, suggesting that many authors, publishers, rights holders, and their representatives are considering blocking their content through both technological and legal means.
In the long term, AIs may have access to a reduced quantity of content for training or, at the very least, content of lower quality. However, an AI trained on lower-quality content would inevitably be less effective and would decline in quality over time.
Conclusion
LLMs have made remarkable progress in terms of quality and their ability to generate content of an acceptable standard for targeted professional use, including tasks inherent to the field of competitive intelligence.
As with any technology, the costs of integration and the time required for professionals to adapt must be factored in and not overlooked. Before realizing a genuine productivity gain, the investment in time and budget can be significant, especially as the costs of these technologies are likely to increase substantially.
Finally, while AI technologies today address compliance needs in intelligence deliverables, this also represents their Achilles’ heel. By leveraging authors’ content to train their models and generate revenue without considering fair compensation upfront, Big Tech and AI developers have drawn ire—similar to what Google News faced in the past. However, unlike Google News, which still drove traffic to news sites, these AIs do not necessarily provide the same benefit.
Bonus
My presentation with Mickaël Réault from Sindup during this Archimag event
(Sorry, but the video is in french)
Presentation deck (sorry in french only…)